Date of Conference: 11 April 2025
比较有价值的点:论文提出了一种新的,低成本的,在离线混部实现方式
1. 问题背景
1) At low loads, most of the GPU memory is allocated but not used, occupying the GPU memory and preventing it from being used by other services;
显存已分配未使用,其他服务也用不了 -> 论文提到了,他们用这个技术来做离线混部。
2) At high loads, due to the GPU memory allocation threshold set by the inference engine, up to 10%-20% of the GPU memory remains unused and idle. Hence, the current GPU memory management is inefficient;
由于推理过程存在不确定性,所以通常会预留一部分显存,导致 10%~20% 的浪费
3) The prefill and decode stages of the inference process have significantly different demands on GPU memory
prefill 和 decode 阶段的显存需求有显著差别(PD分离架构下,P节点不需要存储 kv cache,D节点需要)
2. 解决方案
整体架构
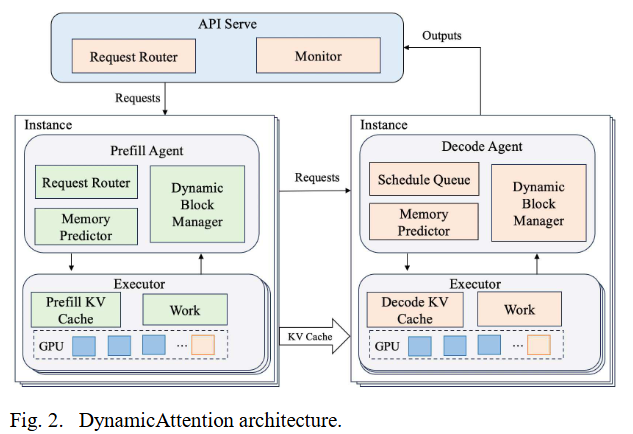
-
Prefill Agent 和 Decode Agent 都有:
-
Queue,管理请求队列,Request Router 和 Schedule Queue 都是一个 Queue
-
Memory Predictor,用来预测内存需求,然后调用 CUDA API 去分配显存
-
Dynamic block manager,应该是用来管理虚拟地址空间的
-
-
Executor:比较类似千帆的 ModelServer
-
KV Cache 就是 kv 缓存,类似我们的 AttentionStore
-
Work 就是推理引擎,负责 token 生成
-